

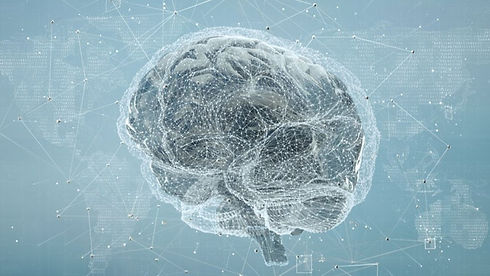
Top publications
2021-2023
[1] Mamalakis M., Banerjee A., Surajit R., et al., Deep multi-metric training: The need of multi-metric curve evaluation to avoid weak learning, Neural Computing and Application, Springer Journals. (Under review)
​
[2] Mamalakis M., Dwivedi K., Sharkey M. et al., A transparent artificial intelligence framework to assess lung disease in pulmonary hypertension. Sci Rep 13, 3812 (2023). https://doi.org/10.1038/s41598-023-30503-4
​
[3] Mamalakis M., Macfarlane S. C., Notley S., et al., Deep multi-attention channels network to detect metastasizing cells in fluoresence microscopy images, arXiv, arXiv:2309.00911, https://doi.org/10.48550/arXiv.2309.00911
​
[4] Mamalakis M., Swift A.J., Vorselaars B., et al., DenResCov-19: A deep transfer learning network for robust automatic classification of COVID-19, pneumonia, and tuberculosis from X-rays,Computerized Medical Imaging and Graphics,Volume 94,2021,102008, ISSN 0895-6111, https://doi.org/10.1016/j.compmedimag.2021.102008
​
[5] Mamalakis M., Garg, P., Nelson, T. et al., MA-SOCRATIS: An automatic pipeline for robust segmentation of the left ventricle and scar. Computerized Medical Imaging and Graphics, 93. 101982. ISSN 0895-6111 https://doi.org/10.1016/j.compmedimag.2021.101982
​
[6] Mamalakis M., Garg, P., Nelson, T. et al., Automatic development of 3D anatomical models of border zone and core scar regions in the left ventricle, Computerized Medical Imaging and Graphics, Volume 103, 2023, 102152, ISSN 0895-6111, https://doi.org/10.1016/j.compmedimag.2022.102152
​
[7] Mamalakis M., Garg, P., Nelson, T. et al., Artificial Intelligence framework with traditional computer vision and deep learning approaches for optimal automatic segmentation of left ventricle with scar, Artificial Intelligence in Medicine, Volume 143, 2023, 102610, ISSN 0933-3657, https://doi.org/10.1016/j.artmed.2023.102610
​
[8] Mamalakis M., Vareilles H., Al-Manea A.,et al., Exploring 3D explainability to uncover patterns in variable sulci recognition., arxiv, arXiv:2309.00903, https://doi.org/10.48550/arXiv.2309.00903
​
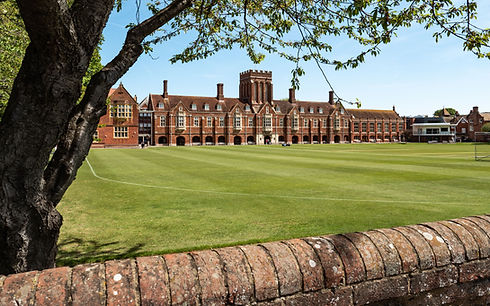
About Dr. Michail (Mike) Mamalakis
A life journey in education
With their dedication to sharing knowledge, Dr. Michail (Mike) Mamalakis received the BSc degree in electrical and computer engineering from the Patras University, Achaia, Greece, the MEng degree in electrical and computer engineering from Patras University, Achaia, Greece, the MSc degree in biomedical engineering from Patras University, Achaia, Greece, and the PhD degree in computer science, artificial intelligence, computer vision, medical image analysis, deep learning, explainability from the University of Sheffield, Sheffield, UK. He was a research associate with the IICD Department, the University of Sheffield from 2021 until 2022. He is a senior research associate with the Psychiatry Department, University of Cambridge since 2022. His research interests include deep learning, machine learning with a particular interest in medical image analysis, computer vision, explainability, uncertainty, new architectures and trustworthy AI.
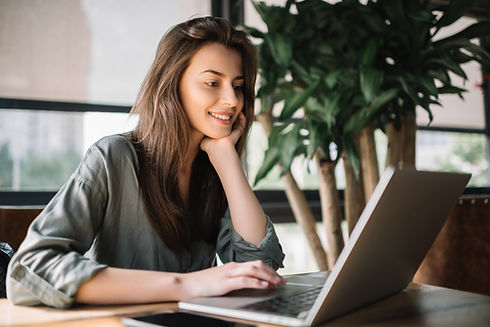